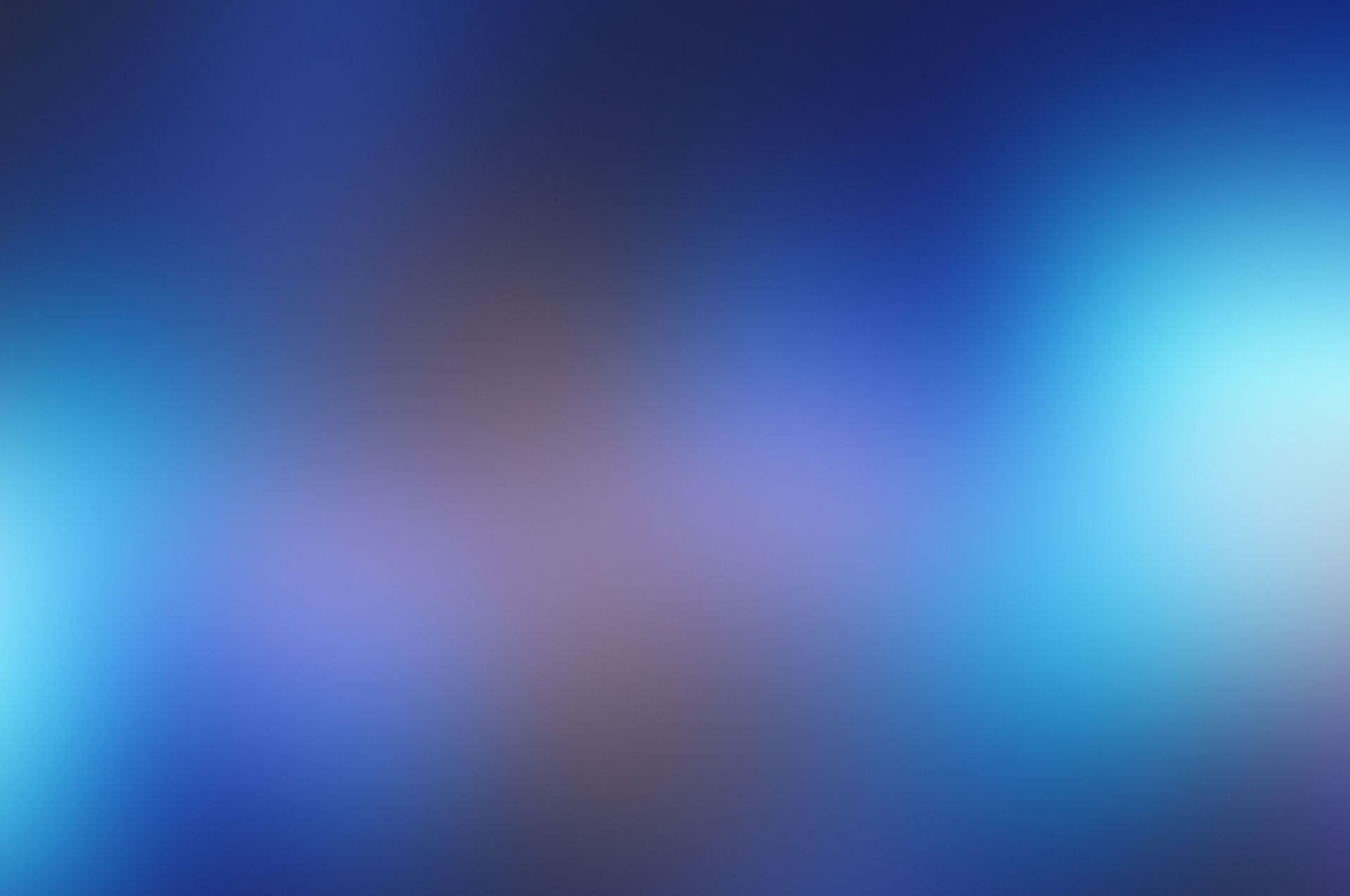
Wellbeing Research Zone: Learning With Children and Young People How to Care Better
Quantitative Research
Quantitative data is all about the numbers. If you are not collecting numbers then you are not doing quantitative research. Quantitative research is less relational that qualitative research as it is often conducted remotely, through surveys with large numbers of people.
​
With Likert Scales then even data that is not numerical can be coded into a numerical value. This is done by introducing categories that somebody filling out the questionnaire would have to select which category they identify with. This might be age categories, ethnicity or eliciting to what extent the person agrees or disagrees with a statement.
​
For example:
​
Homework can cause additional conflict in the home, between parents and children.
​
Strongly Disagree Somewhat Disagree Neither Agree or Disagree Somewhat Agree Strongly Agree
​
The amount of times each category is selected across the whole survey is then analysed through statistical methods to produce a numerical value, so it is possible to claim that 48% of participants strongly agreed with a statement, 33% agreed, which meant overall 81% of participants were in agreement that homework was a source of additional conflict between parents and children based on a sample of 7000 parents of both primary and secondary aged school children.
​
The first step in quantitative research is to identify your sample, which should be representative of the population and large enough to give enough data to avoid errors that are significant with smaller samples. There are different types of sampling to obtain the data you need
Probability Sampling
​
Allows for as much preciseness as is possible.
​
-
Simple Random Sampling
​​
Every person in a population has an equal chance of being chosen
​
-
Stratified Random Sampling
​​
Divides the population as a whole into groups or categories and then selects a random sample from each of those subgroups to ensure equal diversity throughout the sample.
​
-
Systemic Random Sampling
​​
The sample is selected from a list from which the research contacts every nth name in the list.
​
-
Cluster Sampling
​​
Consists of dividing the population as a whole into clusters based on geographical location and then selecting a sample from within those clusters.
Non-Probability Sampling
​
These methods mean there is no way of predicting what probability here is of any member of the population being sampled. This means that the researcher can only draw conclusions within the sample rather than being able to generalise them to the general population.
​
-
Convenience Sampling
​​
How easy the sample is to access, however that does not mean it will be representative
​
-
Purposive Sampling
​​
The researcher selects the sample based on whether the person meets the criteria that is a prerequisite for the study
​
-
QuotA Sampling
​​
Divides the population into categories and then has a set number of people they need from each category.
​
-
SNowball Sampling
​​
The research locates one person who meets the criteria and then uses that person to be able to identify others who meet that criteria. For example, if the researcher was researching the challenges faced by parents of disabled children during COVID19 lockdown, they might use one parent to identify other parents in their network for the researcher to approach.
Strengths
​
-
​Data collected can be very precise, consistent and reliable.
-
Studies can be replicated easily and confirm validity.
-
Relatively easy to analyse.
-
FIndings are more easily generalised if the sampling design is robust
​
Limitations
​
-
Research is remote and difficult to understand the context.
-
Data is simplistic and does not explain complex issues
-
There is a high risk of over-simplistic conclusions being drawn that do not take complex circumstances into account.
-
This can lead to idealistic and ill-informed decisions and policies.